Integrated Business Management – digital transformation
Why bother with advanced analytics?
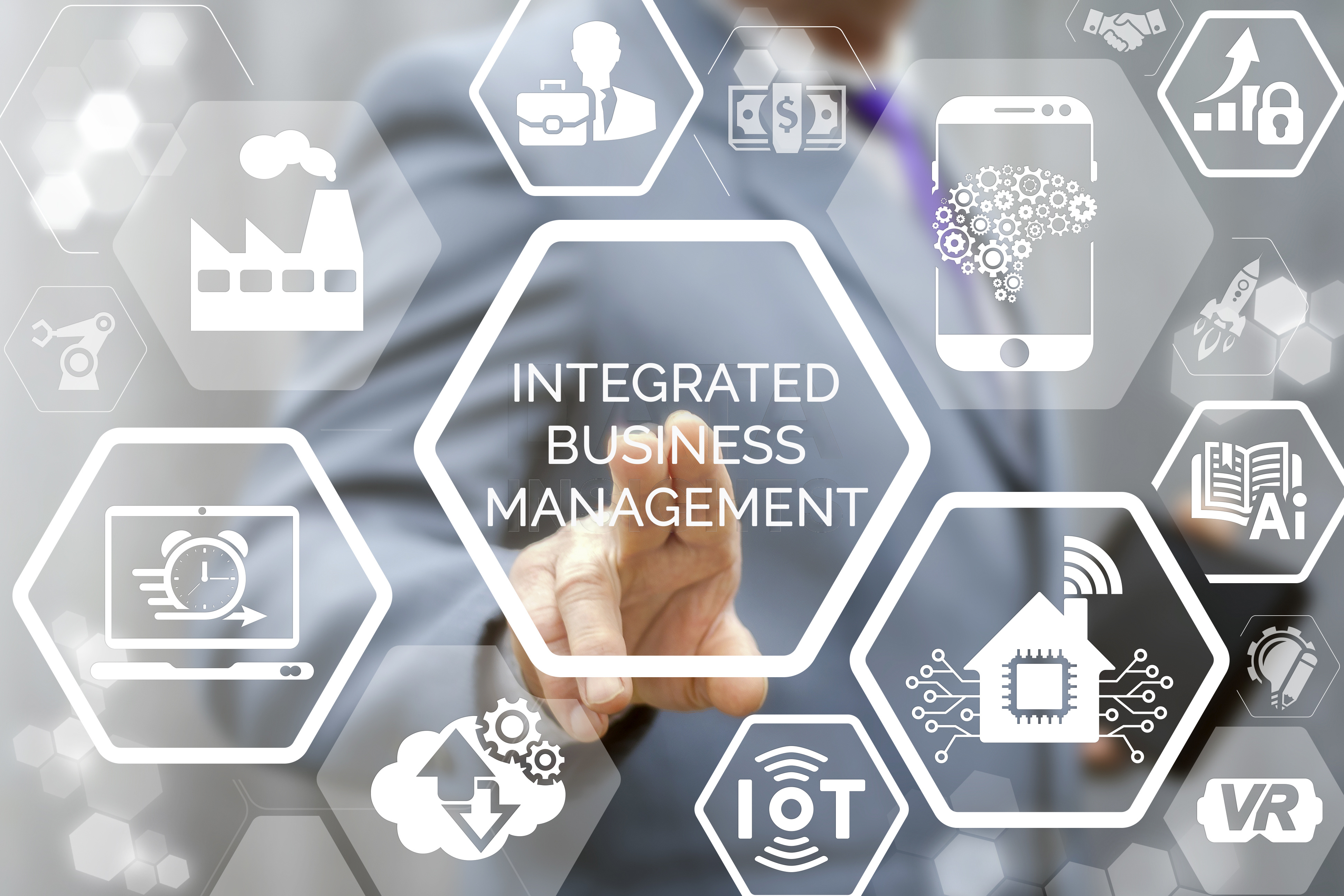
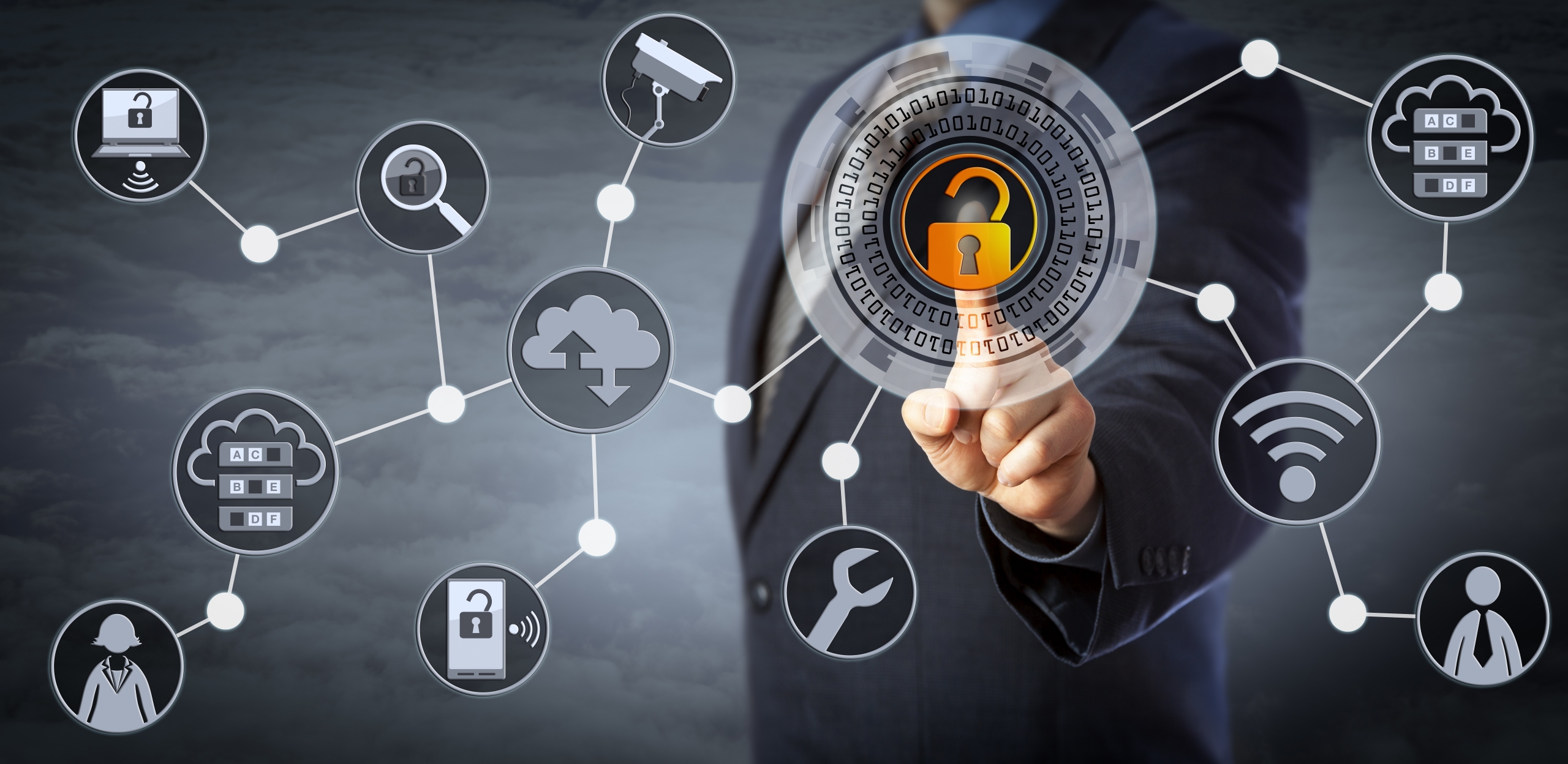
Just 5 years ago systems would not speak to each other without serious effort, usually from a third party, to make the ‘magic’ of collaboration happen. My personal journey with IBM involved excel, SAP BPC, SAP APO, Demand Solutions and various other software solutions to try and get our numbers to be accurate and understood.
Today what we were working on with hundreds of people across many functions can be completed by a small data science team with, I would argue, more accurate results. The advent of cloud storage saw the release of data back to the user because the main barrier to entry for most projects, which is cost, plummeted into the very low thousands instead of millions.
As with all new initiatives it was never quite that simple, but the reality of being able to extract all of your raw data into one place and then transform it to information for the user. Loading it then into visualisation software to create analytics, that would lead to intelligence and action, has been a large part of what many argue is the 4th industrial revolution – digitalisation.
So the many opportunities these changes bring need to be looked at for Integrated Business Management and deployed as commercial offerings where feasible.
The analytical maturity curve
To comprehend how digitalisation will impact the world of integrated business management it is first needed to understand the analytics maturity model. The model shows that as you grow in your digital awareness and abilities your perspective will change from historical to futuristic, from a large percentage of the process involving human intervention to a small to non-existent human interaction and finally from guessing the future to being told what a probabilistic future based on past events and algorithms from a learning computer thousands of times smarter than a human.
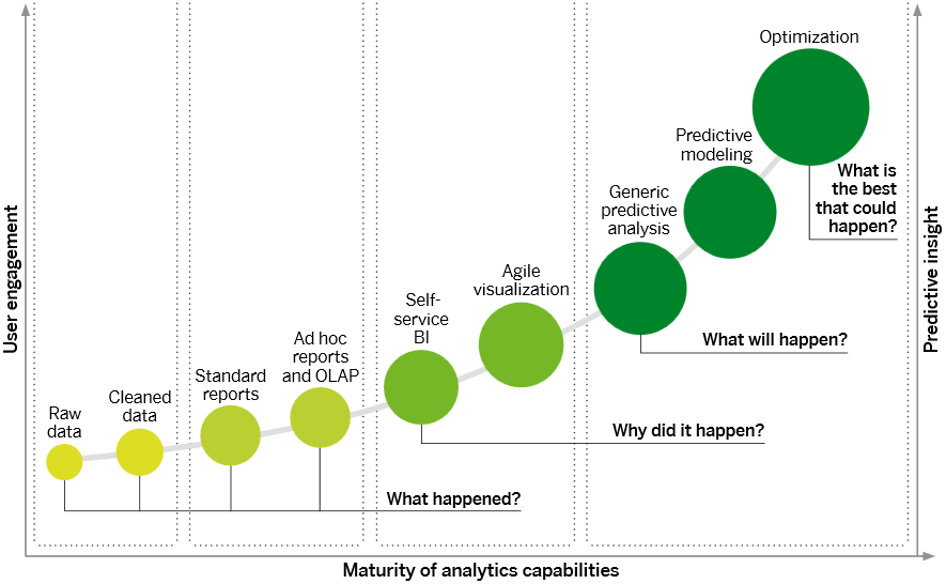
How can advanced analytics help Integrated Business Management?
There are many areas whereby installing standard and advanced analytics can drive the business to faster decisions and actions because this is its main purpose. To give you a flavour of some of the opportunities can bring in adopting a digital approach to IBM can bring I will go through a few examples below:
Management Business Review and Integrated Reconciliation
Utilising cloud services all of the raw data can be accessible to two areas to help drive decisions and actions in the IR and MBR, these being:
1) Standard analytics can utilise visualisation tools (e.g. Tableau and Power BI) to pull from transformed information and intelligence to build the decision making tools that will allow leaders to make decisions on the fly. My team built a strategy tool that could drill down as far as the user wanted to go to become comfortable with the data and then utilise it’s flexibility to highlight to the C-Suite the issues the business were facing and make recommendations on the way forward in line with the new strategy. As we had all the data the tool took a week to build and was utilised in a 2 day workshop by a select team.
Getting the right data into the right quality and transforming it to information that supports the strategy at all levels (strategic, tactical and operational) allow you to then visualise the data various different ways.
2) Utilising the data from the cloud you can create a small, circa 5-10 individuals, data science team that will starting looking at building what-if scenarios and giving the scenarios probabilities of success. I worked alongside AT Kearney to build our first data science team that looked at the probabilities of product portfolio optimisation with a target value in the hundreds of millions. This same methodology can be applied to IR and MBR for probability of risks materialising and/or opportunities being won.
Eventually you would also look at probability of launch success in the PMR and replacing the DMR teams – see below for more details.
Product Management Review
Media companies have for several years now employed data science teams to ‘run the numbers’ on stories they want to publish. Utilising the success of past stories and algorithms to match profiles of stories and users the data science teams could predict whether a future story would be well received and sell more papers/magazines or not be as well received. As time went on the data science teams became increasing more accurate as they adjusted their models and enhanced their algorithms.
We can take this example and apply it to the PMR. My experience of sitting on the PMR was listening to people who wanted investment create their own data and scenarios and presented it back to the group. This caused problems on three fronts, namely that the better the numbers the greater chance they were going to receive that investment; the numbers they gave were not verified and they were not from an independent source.
Implementing a data science team methodology allows for an independent view of the potential success of new product launches and trade-ups.
Demand Management Review
Advanced analytics such as machine learning and robotics process automation rely on numerous transactions and programming to turn historical data into future allocations and predictions. Much the same as statistical forecasting, machine learning will work with the data and experts, such as demand managers, to start learning the algorithms and patterns to predict future outcomes. We started deploying machine learning in another part of the business on our financial forecast, the test showed an increase in accuracy to 95% and so they started rolling it out across the globe.
Utilising current technologies you could build a machine learning algorithm to derive a forecast of high accuracy and remove a majority of demand management teams. The data can then be built into visualisations that can be interacted with sales teams to help provide indicators of change.
Supply Management Review
Following on from the DMR, robotics process automation can take the data and allocate it automatically to create the supply plan adherence schedule. It can use machine learning to allocate safety stock levels and provide suppliers with requests for raw materials. As AI grows you can then start deploying
The data science can look at risks and opportunities to provide further insight to the SMR, with standard analytics bringing it all together in visualisations for the management team.
Summary
Many organisations are embracing the digital transformation with increased demand for Chief Data Officer’s and utilisation of data becoming part of the normal course of business in this fast changing environments. Many businesses, including those from a data background, are having to change the way they operate to take advantage and stay relevant in this new digital world. I
When I started out on the journey of IBM it would have been of great advantage if their could have been a digital offer alongside the process as it slowed us down greatly in deployment as we designed it, learnt from it and built it. As we were also learning how to benefit from IBM.
In conclusion, it is my belief, that IBM can seize the opportunity to integrate their process with the data and introduce a new offer to build the data models, visualisations and teams to drive a digital IBM. This will allow IBM to remain current with changing practices and reliance on data and help businesses get to value more quickly, enhancing the partnership.